The Myth of Machine Learning
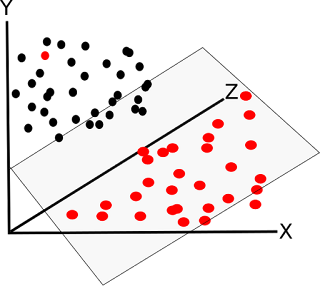
Recently there has been a surge in the number of companies claiming machine learning capabilities, from startups to large organisations. The media is full of "machine learning" claims, investors seem to be dropping large amounts into startups claiming to have "machine learning" or "artificial intelligence" capabilities. From Logo design companies to delivery companies, they all claim to have implemented "machine learning". I recently saw a press release for a US based app development company that had raised a significant 7 figure sum, claiming they had developed "Human assisted machine learning" ! One has to ask, what is that ? Any machine "learning" has to be assisted by humans anyway, who would configure the algorithms, applications and hardware, not to mention the training ? Neural Networks and AI genetic algorithms have been around for a long time. So why now ? The answer is data (and to some extent computing power).